Building Toward Virtual Cells
We aim to accelerate science by improving access to centralized AI resources for developing, fine-tuning and using state-of-the-art cell biology models.
Transcriptomic Models & Datasets
Find and easily access AI resources that can help provide insights into patterns of gene expression across cell types, cell states and species.
Featured use case
Predict Cross-Species Cell Types With TranscriptFormer
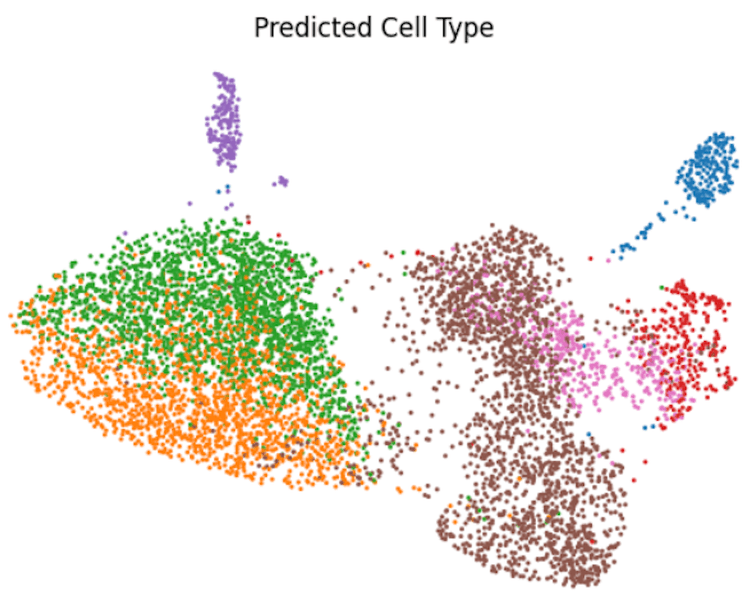
TranscriptFormer is a family of generative models representing a cross-species cell atlas trained on up to 112 million cells spanning 1.53 billion years of evolution across 12 species. It jointly models genes and transcripts using a novel generative architecture, enabling it to function as a virtual instrument for exploring cellular biology.
Learn how to use TranscriptFormer to generate embeddings and assign cross-species cell type annotations with this step-by-step tutorial.
Explore Transcriptomic Models
scGenePT
A collection of single-cell models for perturbation prediction that leverages the scGPT foundation model for scRNAseq data by injecting language embeddings at the gene level into the model architecture.
scGPT
A foundation model designed to integrate and analyze large-scale, single-cell multi-omics data using a generative pre-trained transformer (GPT) architecture.
scVI
A probabilistic deep generative model designed to analyze single-cell RNA sequencing (scRNA-seq) data.
Explore Transcriptomic Datasets
CZ CELLxGENE Discover Census |
source
This dataset is a large-scale scRNA-seq resource that integrates data from over 33 million human cells across various tissues, conditions and disease states from human and mouse datasets.
Adamson et al. |
source
This dataset features single-cell RNA-seq profiles from Perturb-seq, capturing UPR responses to single and combinatorial CRISPR perturbations of ER homeostasis in K562 cells.
Norman et al. |
source
This single-cell RNA-seq dataset was generated by combining Perturb-seq with a CRISPR activation library to systematically map gain-of-function genetic interactions in K562 cells.
Imaging Models & Datasets
Discover AI resources that can help provide insights into cell types and states by modeling their morphology and cellular organization. Get started using models and data faster.
Featured use case
Examine Protein Localization Changes With SubCell
SubCell is a collection of self-supervised Vision Transformer (ViT) models designed to analyze cell phenotypes and subcellular protein distribution from microscope images.
Learn how to use SubCell to examine protein localization changes following SARS-CoV2 infection. We provide a step-by-step tutorial to quickly get it running on your local environment, plus pre-processed data for model inference that requires no transformation.
Explore Imaging Models
CELL-Diff
A diffusion transformer model designed to generate detailed protein localization images from protein sequences (“sequence-to-image”) or output protein sequences based on microscopy images depicting protein localization (“image-to-sequence”).
SubCell
A collection of ViT models that was pre-trained on single-cell fluorescence microscopy images from the Human Protein Atlas (HPA) and generates feature embeddings that encode the protein localization patterns.
Explore Imaging Datasets
Human Protein Atlas |
source
Images that capture the subcellular localization of proteins relative to other cellular components and their variation across single cells and different cell lines. Proteins from 13,147 genes were imaged.
Human Protein Atlas for SubCell |
processed
Contains cropped images from the Human Protein Atlas subcellular section that were generated to train the SubCell models. The original HPA images were segmented to identify cells and crops were made centering around each identified cell.
OpenCell for SubCell |
processed
This dataset was created to evaluate the SubCell model using images from OpenCell. The maximum-intensity z-projection images were cropped, centering around each nucleus.
Accelerating Model Development and Use for Biology
Discover curated cell biology models and datasets
We’re aiming to build a one-stop platform for models and datasets that our team has vetted for usability – starting with select models and datasets in imaging and transcriptomics.
Find high-quality, ML-ready data to accelerate model development
Access large, processed datasets for model training and validation that minimize the need for data wrangling and transformation.
Run a model faster using notebooks
Each model comes with a notebook to help you get it running quickly using demo data with minimal debugging.
Gain use of and feedback on your model
Reach an engaged audience to apply your models and provide feedback to improve their performance for biological use cases.
Our Approach
Accelerating Biology With AI
Over the next decade, the Chan Zuckerberg Initiative is focused on understanding the mysteries of the cell, which we will achieve through major investments in data, models and applications.
We started this effort by building and providing access to high-quality, standardized biological data on platforms like CZ CELLxGENE and the CryoET Data Portal. We then established one of the world’s largest computing systems for nonprofit life sciences research. We’re using this system to build virtual cell models that can predict the behavior of healthy and diseased cells, which will have broad applications for biomedical research, disease diagnosis and therapeutic development — bringing us closer to CZI’s mission to cure, prevent or manage all diseases by the end of this century.
We intend to work with the scientific community over the next several years to build these virtual cell models. Releasing this platform is the next step in that journey. We will demonstrate our commitment to openly sharing resources for modeling, evaluating and analysis of cellular data with the scientific community on this platform.
We’re prototyping in the open and making early models, datasets and this platform available for the scientific community for early access use and feedback.
Building Better Benchmarks
We’re bringing together the AI and biology communities to discuss challenges, opportunities and ways forward to improve model benchmarking. Read more about our efforts below.
12.09.24
Biological Bias Assessment Guide for AI in Biology
Check out the Biological Bias Assessment Guide — a compilation of resources to offer a framework for identifying and addressing biases in AI models for biology, with the potential to empower interdisciplinary teams to build more reliable, inclusive tools across diverse biological applications.
12.09.24
Designing a ML Competition for CryoET Data With Limited Annotations
Take a behind-the-scenes look at Chan Zuckerberg Institute for Advanced Biological Imaging's efforts in the development and hosting of a groundbreaking ML competition to boost cryo-electron tomography (cryoET) particle detection, challenging models to excel with minimal annotations and showcasing a unique experimental dataset.
12.09.24
Insights From the CZI-hosted Benchmarking and Evaluation Workshop
Learn about insights from a recent CZI workshop on building robust benchmarking for AI in biology, highlighting the importance of reproducibility, high-quality data and community collaboration to drive scientific progress and innovation in biological modeling.
News & Stories
Interested in learning more about our work on the virtual cell models platform? Get the latest information from the links below.
Sign Up for Our Mailing List
Join our mailing list to stay updated on the latest news, collaboration opportunities, funding announcements and more. You can unsubscribe at any time.